How to Estimate Probabilities for Trading | Crash Course
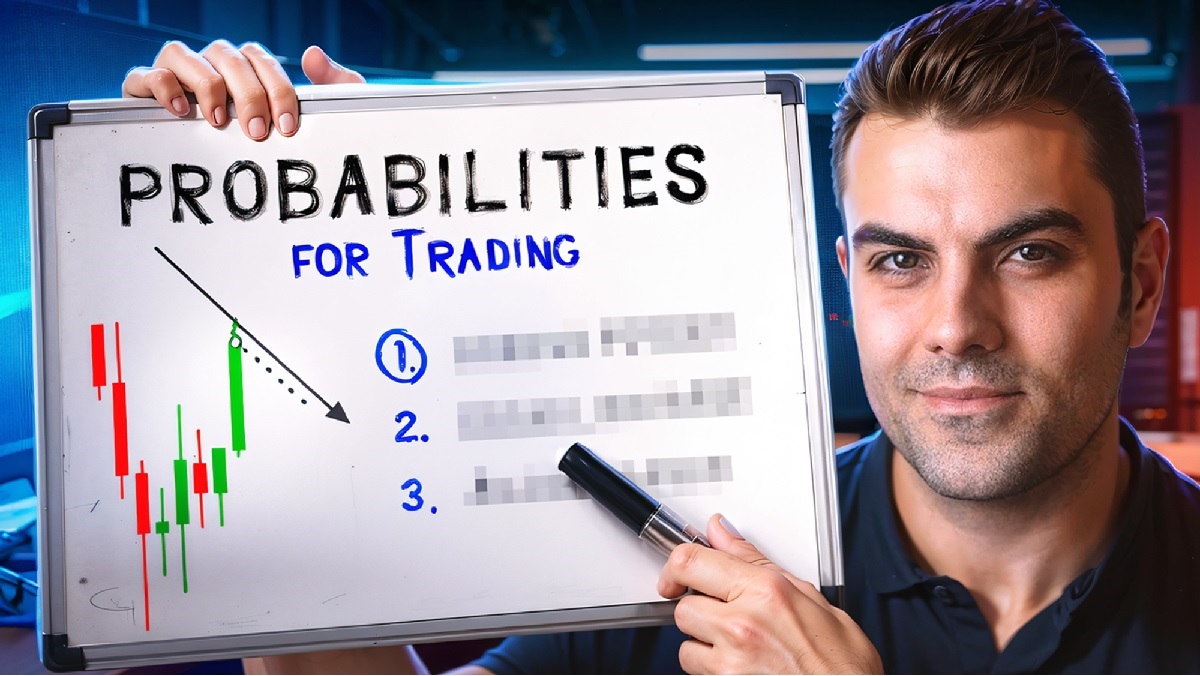
You're probably familiar with the saying, "Trading is a game of probabilities." In fact, it's become a bit of a cliche online; a comfort blanket for traders dealing with losses.
I agree that trading is all about probabilities. But if that's the case, why do so few traders include probabilities as part of their analysis or decision-making?
There are two main reasons for this:
- Most traders don’t know how to estimate probabilities
- Subconsciously they’re afraid that estimating probabilities will reveal most of their decisions are actually based on luck.
But if you’re serious about trading, estimating probabilities is a skill you need to develop. So in this guide, I'm going to give you a crash course of what you need to know to get started.
Now, you might notice I just referred to estimating probabilities as a skill. It is indeed a skill, and quite a complex one. That means, it's something that needs to be learned and developed; it's not something you'll be great at just by reading this guide.
Many traders will be put off by that fact. Learning to trade is enough skill development on its own, why would you want to start learning another complex skill on top of that?
Well, the truth is, estimating probabilities is so fundamental to trading that you significantly reduce your chances of succeeding by neglecting it.
In fact, whether you make estimating probabilities a conscious activity or not, it's still playing a part in your trading. Throughout our everyday lives, each time we make decisions or judgements we're estimating probabilities.
When you decide whether to open or close a trade, or any other trading action, you're subconsciously making a judgement about the likelihood of something happening.
But what if your judgements are inaccurate? How would you know if you haven't made the process a conscious one? How can you improve your accuracy if you're not measuring it?
Additionally, estimating probabilities solves many trading psychology issues. It improves your confidence and stops you hesitating about decisions. It allows you to structure your system in a more meaningful and effective way.
Ultimately, if trading is a serious pursuit for you, you need to focus on developing this skill.
I'll outline some of the key things you need to understand in this guide, but please keep in mind that this is just the starting point. If you want more extensive training in probabilities that's tied to an effective method of trading, you should join our Trader Development Program. If you haven't already watched our free Masterclass and read the follow-up emails, that would be a great place to start.
Now, let's begin...
Putting Probabilities in Perspective
Let's start by changing our perspective of the world we live in.
As Benjamin Franklin famously said, “in this world nothing can be said to be certain, except death and taxes.” We always have to assign a non-zero probability to things, as nothing is ever absolutely certain.
But humans don't handle probabilities well intuitively. In fact, even when given a probabilistic forecast, we often struggle to comprehend it.
Imagine you check the weather today, and the forecast predicts a 70% chance of rain. How would you interpret that?
For some, this might suggest that it's going to rain for 70% of the day. Others might interpret it as 70% of forecasters believe it will rain, while a few might assume it represents that 70% of a given location will experience rain.
In reality, a 70% chance of rain means that over 100 days—each forecasted with a 70% chance of rain—it should rain on approximately 70 of them.
This goes against our natural inclinations. We tend to simplify forecasts by thinking something will or won't happen (or maybe it will happen). We don't usually consider a percentage probability, like a 70% chance of rain.
Instead, when faced with a percentage probability, we fall for the 'wrong side of maybe' fallacy—meaning that we presume the outcome indicated by the side of 50% the forecast leans towards.
So if there’s a 70% chance of rain, we assume it's going to rain. If it doesn’t, we think the forecast was inaccurate because it’s on the wrong side of maybe. On the other hand, if it does rain, we see the forecast as accurate.
We apply this flawed logic to our entire lives. Since we only experience one reality, we find it incredibly difficult to imagine that anything other than what happened could have occurred.
However, as Nassim Taleb, the mathematician, best-selling author and former derivatives trader explains, we are simply living in one outcome that surfaced from a vast number of potentially possible outcomes.
Just as the future holds many possibilities, the same was true in the past. Among many potential outcomes, each with their own probabilities of happening, we're living in the one that did occur. The others can be thought of as what Taleb refers to as ‘alternative histories’.

With this in mind, when something takes place in the markets, we have to resist considering it the ‘right outcome’. Instead, we should view it as one of many 'alternative histories', each with its own probability of occurring.
Why We Need to Be Precise With Probabilities
In 1948, psychologist Bertram Forer requested his 39 psychology students to take part in a personality test. They were told they'd each receive a short personality profile based on their responses.
A week later, Forer provided the students with their personality profiles and asked for their opinions on the accuracy of them. Impressively, the profiles were judged to be highly accurate. The students, on average, evaluated the accuracy at 4.3 out of 5 stars.
The students didn’t know, however, that they all received the same personality summary. The document included 13 vague, generalised phrases taken from an astrology book for horoscope creation.
What the students experienced is now recognised as the Forer Effect, a psychological predisposition to interpret broad descriptions as an accurate assessment tailor-made for themselves. But in reality, they're vague and generalised enough to relate to anyone.
But more importantly for us, it shows us the pitfalls of using vague language with our analysis and forecasts. As Philip Tetlock, an expert on superforecasters, observes, vague language is elastic language. We can make it fit what we want it to fit.
This was demonstrated in a study the CIA performed on their intelligence analysts. They were evaluating 'words of estimative probability'. In other words, phrases such as “very likely” or “there’s a good chance” to express the likelihood of an outcome. The goal was to identify what percentage probability their analysts attributed to these expressions.
For example, suggesting something is “quite likely to happen tomorrow”. Does that indicate there’s a 51% likelihood, or a 99% likelihood? That’s a big difference and would greatly affect your decision-making.
The results were shocking. Remarkably, these CIA analysts who make judgements regarding future events for a living, some even influencing decisions about whether to initiate war, showed terrible accuracy.
The diagram below shows the range of different answers for each statement:

What this tells us for our trading is that the only way we can see how accurate we are at forecasting, and improve our ability, is to make sure our forecasts contain no ambiguity – they must be exact.
Not only do they need to be precise in terms of the specific probability assigned, but also when we anticipate the event to happen by.
In other words, we have to break the rule analysts in the financial media abide by. They state what's going to happen, or when something is going to happen, but never both. That way, they can always claim they were correct - how convenient!
You'll also see trading gurus do this when they make claims like, "EUR/USD is going up". No matter when it happens, they can remind you of their 'correct' prediction.
However, in our trading, we don't want to deceive ourselves. We need to be accurate with our predictions for two reasons:
- To help our decision-making (e.g. is this a viable opportunity or not?)
- To measure and improve our accuracy (therefore improving our ability to assess opportunities in future)
Therefore, we need to assign a percentage probability and a deadline to our estimates. This deadline can be time-based, or simply based on the next prices moves (e.g. first-order outcomes, second-order outcomes, and so on. This is something we discuss in more detail inside the Trader Development Program).
Estimating Probabilities Precisely
When it comes to estimating probabilities, our aim is to eventually become as precise as possible.
However, when you first start estimating probabilities it may be difficult to be extremely refined. Therefore, it's a good idea to begin by estimating with a broad stroke and, as you gain experience, start becoming more and more defined.
Initially you may want to assign probabilities in sets of 25%.
In other words, 0, 25, 50, 75, or 100% probability.
Then, as you gain experience, you can refine this to sets of 10% (0, 10, 20, 30, 40, 50%, and so on).
The objective, however, is to reach a point where you think in even more refined terms, so instead of 30%, it might be 32% or 33%. The lower the unit you can allocate to probability, the better, provided it truly represents a genuine differentiation. Therefore, if you designate a 43% probability to something, those instances really should happen 1% more frequently than a 42% outcome.
These minor variances in probabilities might seem unimportant, but they can have important consequences when evaluating different trading opportunities.
It might seem crazy to be so precise with probabilities, perhaps even impossible. But by the end of this guide, you'll understand more about the process that will lead you to making such precise forecasts.
Psychologist Barbara Mellers conducted tests on the superforecasters at the Good Judgment Project, who tend to use a meticulous forecasting approach. She assessed how their accuracy would change if she rounded their percentages to the closest 5%, instead of the precise units they were using. The results showed a reduction in their accuracy due to rounding, therefore demonstrating that their precise numbers truly increased their accuracy.
Steps For Estimating Probabilities
Let’s start with a question. An individual has been described by their neighbour in the following way:
“Steve is very shy and withdrawn, invariably helpful but with little interest in people or in the world of reality. A meek and tidy soul, he has a need for order and structure, and a passion for detail.”
Question: Do you think Steve is more likely to be a librarian or a farmer?
Daniel Kahneman asked this question to participants as part of a research study. As you might anticipate, most responded that Steve is probably a librarian.
But, this isn't the best method to estimate probabilities.
If you answered 'librarian,' you're concentrating on the specific characteristics and matching those to occupational stereotypes. However, there's a more critical piece of information that would lead to a better response to this question...
How many farmers are there in the population compared to librarians?
Kahneman estimated that the ratio approximately equals 20 to 1. So let's use that for our initial guess.
Let's picture having 2,000 farmers and 100 librarians. How many members of each group align with Steve's description?
We could presume a more significant proportion of librarians match the description. For instance, maybe it's 30% of librarians and just 5% of farmers.
Therefore, 30% out of 100 equals 30 individuals, but 5% of 2,000 equals 100 individuals.
So, reconsidering the initial question: is Steve more likely to be a librarian or a farmer? Clearly, he's more likely to be a farmer.
What I’ve basically just described is Bayes' Theorem or Bayes’ Rule, a method enabling us to assess a probability by starting with an initial broad probability (known as a prior, or base rate) and updating it with new information based on the uniqueness of the situation.
Here's the explanation of the formula from Wikipedia, but don't worry if it seems complex, we can take a more straightforward approach:

So let's break things down in a more simple way that we can apply to our trading. We'll start by going through the 'Steve' example again.
We knew there was a ratio of 20 farmers to every librarian. This is referred to as our prior (or base rate). So, if we were to simply speculate whether Steve was a farmer or a librarian, considering only the population, we'd guess there’s a 95% probability he’s a farmer.
But we have additional details. Therefore, we can adjust our probability considering that additional evidence and suggest there's now a 77% probability that he's a farmer. This result is referred to as the posterior.
Every time we update our probability, that will become the new prior which we would then update if new information or conditions emerge. This would allow us to reach a new posterior probability.
As we receive new information, we can continually update the probability in proportion to the weight of the evidence. By going through this fine-tuning process, we gradually move closer towards the truth. Interestingly, this is similar to how we intuitively calculate probabilities too, which we'll discuss a bit later.
Applying This to Our Trading
There’s a sequence of steps you can follow which has been proven to improve your forecasting accuracy. I'm going to explain this sequence in quite a broad way so it can be applied to any method of trading, however, for the Duomo Method we cover specific steps inside the Duomo Trader Development Program.
1. Fermi-ise the question
This first step is more relevant to fundamental analysis and may not be particularly relevant for your method of technical analysis.
This step is based on Fermi problems, named after Enrico Fermi. This is a category of problems that require estimations and assumptions to solve. They're usually creative or out-of-the-box questions, such as "how many piano tuners are there in Chicago?"
It's unlikely anyone knows the answer specifically, but they can get to a closer approximation by thinking about aspects of the question they know, like how many people in Chicago, how many of those people might have a piano, and how often might a piano need tuning.
So you’re breaking things down to ask yourself questions like "what would have to be true for this to happen?" or "what information would allow me to answer the question?"
This is a good approach to forecasting. We are still guessing overall, but we're going through a process and questioning it to get that guess. It’s going to be a better guess as a result of that.
By using Fermi's method of breaking a question into components, we can distinguish between the known and the unknown, and heavily scrutinise any assumptions we’re making.
2. Outside view
Now it's time to start the process we discussed a moment ago, using Bayes' Rule. You'll remember that we start with the prior or base rate.
Another name for this, as outlined by Daniel Kahneman, is the 'outside view'. We then also have the ‘inside view’.
The inside view is when you make predictions based on your understanding or opinion of the details of something, whereas the outside view is when you ignore those details and use an estimate based on other cases that are roughly similar.
For example, when deciding if the person was a Librarian or a Farmer earlier, the outside view would be to look at the number of people who are farmers or librarians. The inside view is to think about which personality characteristics are more suited to one or the other.
It’s important that we start with the outside view first. This is because we usually make estimates by starting with a number and adjusting from there. That number we start with is known as the ‘anchor’.
We have a tendency to under-adjust from the anchor, so if we have a bad anchor, we’ll have an even worse estimate overall.
So we start with the outside view, also known as the base rate, or in Bayes rule - the prior.
For our technical analysis, there are two approaches we can use for this. An informed approach and an uninformed approach.
Informed approach:
If the scenario is something that happens frequently and we have data about how often particular outcomes happen, we may use that as our 'outside view'.
For example, if there are specific criteria that indicate a particular setup for you and you're estimating the probability of Price Move A vs. Price Move B, you may use previous data as your base rate.
Uninformed approach:
If you have no previous data, your outside view might be based on the probabilities if the markets were completely random and you had no reason to favour one price move over another.
For example, in the below screenshot, Price Move A is 60 pips and Price Move B is 30 pips. Using an uninformed approach, we can assume Price Move B is twice as likely to occur as Price Move A.
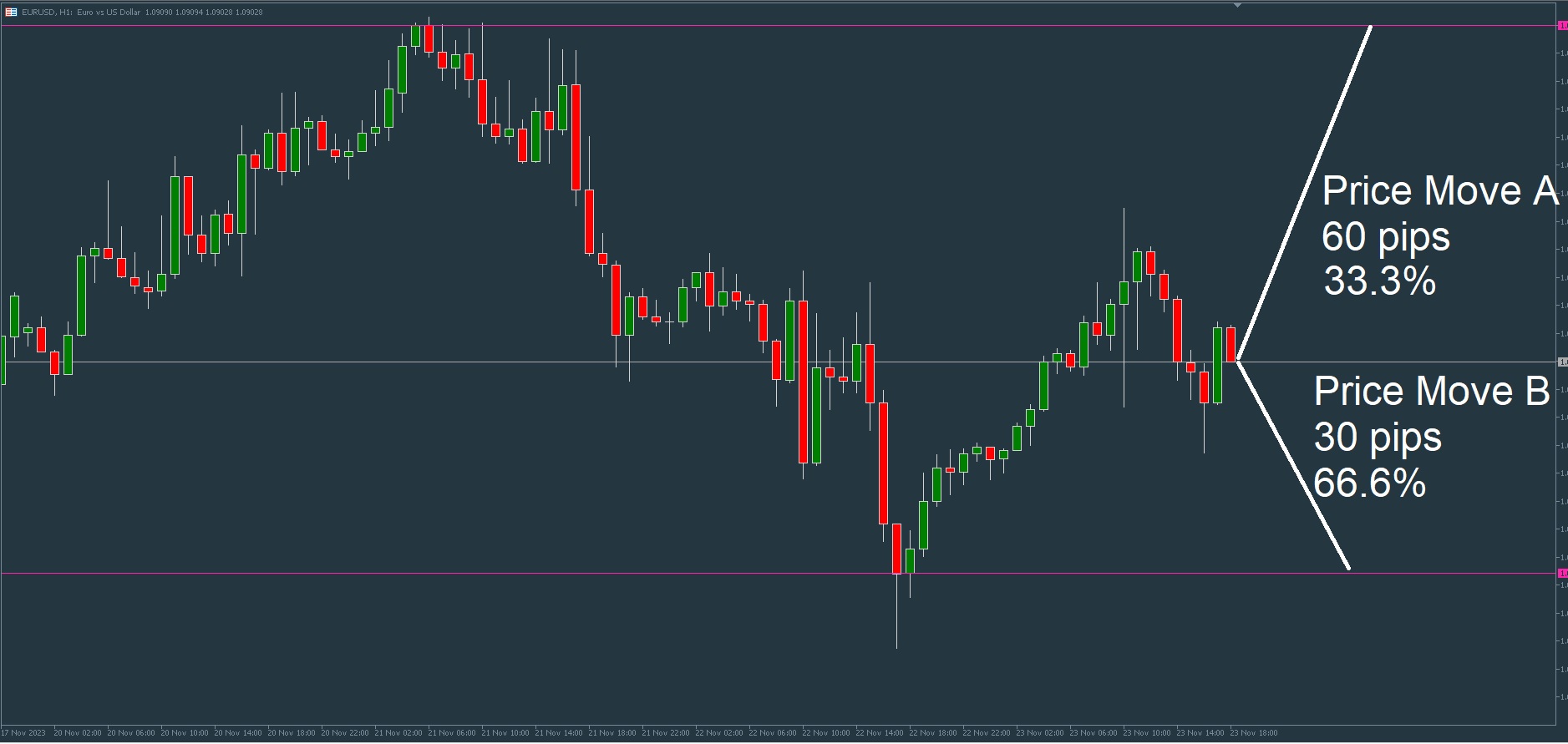
As we'll discuss more later, the uninformed approach is fine to use when you're just getting started, but you should aim to replace it with the informed approach as soon as possible.
3. Inside view
Once we’ve got our starting point from our outside view, we can then move onto the inside view. This is where we’re looking at the uniqueness of that particular situation to adjust the probabilities to a certain extent.
Thinking back to Bayes’ Rule, here we’re constantly updating our priors. A description I’ve heard which I love for this is that we’re constantly using snowflakes of evidence. Each individual snowflake might not change our prediction much. Just like a snowflake covering the ground, it’s not going to cover much. But as you get more and more snowflakes, it can completely change the situation.
In terms of our technical analysis, this is where you will need to assess the context of the market leading up to that point in time. The aspects that you consider to be important for the context will depend on the particular method of trading you're using and how you analyse the markets. Needless to say, the method you use will greatly determine how precise your probability estimates are. With the Duomo Method, we are able to achieve incredible accuracy.
To give you some guidance, here are some factors you may want to consider:
- Volume of recent price moves
- Liquidity around current price (when it's possible to use this information - check out our guide here on how markets operate)
- Momentum of recent price moves
- Overall trend (short, medium, and long term)
- Previous volume, liquidity, and momentum in comparison to recent moves
- Previous volume, liquidity, and momentum previously at the same price level
- Further paths of resistance
- Strength of your setup
- Dependability of your setup
- Upcoming market moving events
- Previous market moving events
- Fundamental analysis
Each of the factors you consider from the uniqueness of the situation can be used to make slight changes to the probabilities. Remember, the size of the changes should be weighted according to how relevant or important the information is, and how reliable it is.
4. Other opinions
This step is only relevant for you if you have contact with other traders (such as our community) or use sources of information that influence your trading decisions.
Only once you've estimated your probabilities following the steps outlined above should you consult others for their opinions. When you do this, you should be looking at similarities and differences with your own view, and making sure you don’t under or overreact to any of this information. In particular, look at experts in the field and any prediction markets, since those will allow you to tap into the wisdom of the crowd.
You then can synthesise all these views into one single view. And at that point, you want to express your judgment as precisely as you can, using a very precise scale (e.g. 32% rather than 30%).
5. Updating your view
Traders have a habit of doing their analysis and then leaving it alone, or even being stubborn and refusing to believe anything could change or be wrong.
However, the world is dynamic and so are the markets; things are always changing. New information may come to light.
It's important to not be stubborn in your views or estimates. You need to have, as the famous forecaster Paul Saffo says, "strong opinions, weakly held."
Improving Your Ability to Estimate Probabilities
As I mentioned at the start of this guide, estimating probabilities is a skill. Therefore, the first step to improve your ability is, quite simply, to practice doing it. Like other skills, mastering it isn't going to happen just by theoretical reading or explanations; you need hands-on practice.
Of course, you're unlikely to be very good at first. But you need to try anyway so you can start building the implicit knowledge. That's what practice is for!
However, not all practice is good practice. Philip Tetlock compares it to practicing free throws in basketball.
You could spend hours practicing free throws every day for a year, and assume you're amazing at them. But, if you were practicing in the dark, your skills are unlikely to have improved at all. You'd be lacking a fundamental part of good practice... feedback.
With that in mind, it's important that you collect data about your estimates and what outcome ended up occurring. This will allow you to gauge your average level of accuracy over time and set out changes for improvements.
You can do this through a process known as calibration. We won't be discussing that in this guide, but it's covered in detail in our Trader Development Program and has led to remarkable results from our members.
The next step for improving your estimates comes down to data collection.
During the '90s, Joshua Tenenbaum, a cognitive science professor at MIT, conducted extensive studies examining our everyday, casual prediction methods.
Tenenbaum concluded that, impressively, we can make highly accurate predictions based on minimal information and adapt them as we consume more data through our experiences. Subconsciously, we use a process similar to what we've been discussing. This cognitive method is referred to as Bayesian cognition.
However, Tenenbaum found an important aspect that dictated how accurate the predictions were. It didn't matter how great the process was for making adjustments to the probability, if the base rate was incorrect the estimate was inaccurate. Therefore, the most critical thing we need to improve is our base rates / priors.
The best way to do this is to start collecting data. Start by collecting data for base rates, and then begin collecting data on contextual elements that define the uniqueness of a situation.
The quickest way to do this is to make use of a trading simulator. You can find my recommended simulator here (use code DUOM10 for an additional 10% discount).
Wrapping Things Up
Forecasting the probabilities of future events is a complex skill and there are many other things we could discuss in this guide. For example, we haven't discussed how biases influence our judgements, or what personality traits and abilities have been shown in studies to be optimal for this process.
If you're interested in learning all of this in more detail, we cover it extensively in our Trader Development Program. If you haven't already gone through our FREE Masterclass and follow-up emails, that would be a great place to start.
This is a process that can be done in either a simple or complex way. Regardless of how you choose to approach it, it will be much better for your trading performance than not estimating probabilities at all.
In the end, whether you choose to make this a conscious process or not, you'll still be doing it subconsciously anyway when you make decisions in the markets. Therefore, it's much better to turn it into a conscious process, start measuring it, and work on improving it. Then you can stop leaving your decision-making to chance.